Self-Supervised Intensity-Event Stereo Matching
Abstract
Event cameras are novel bio-inspired vision sensors that output pixel-level intensity changes in microsecond accuracy with a high dynamic range and low power consumption. Despite these advantages, event cameras cannot be directly applied to computational imaging tasks due to the inability to obtain high-quality intensity and events simultaneously. This paper aims to connect a standalone event camera and a modern intensity camera so that the applications can take advantage of both two sensors. We establish this connection through a multi-modal stereo matching task. We first convert events to a reconstructed image and extend the existing stereo networks to this multi-modality condition. We propose a self-supervised method to train the multi-modal stereo network without using ground truth disparity data. The structure loss calculated on image gradients is used to enable self-supervised learning on such multi-modal data. Exploiting the internal stereo constraint between views with different modalities, we introduce general stereo loss functions, including disparity cross-consistency loss and internal disparity loss, leading to improved performance and robustness compared to existing approaches. The experiments demonstrate the effectiveness of the proposed method, especially the proposed general stereo loss functions, on both synthetic and real datasets. At last, we shed light on employing the aligned events and intensity images in downstream tasks, e.g., video interpolation application
Result
Results of different methods on synthetic dataset. Monocular model does not work well as
it cannot be fine-tuned under this setting. Stereo matching between intensity and reconstruction
fails because the color discrepancy prevents the network to relate corresponding pixels. After selfsupervised training, our predictions are on par with the ground truth. The reconstruction network
is FireNet and the stereo network is the modified AANet.
Method
Our self-supervused stereo system follows a few steps: First, we convert event stream to reconstructed images via off-the-shelf models such as FireNet or E2VID, so that we have an image pair, with one intensity image and one reconstructed image. Second, We train the system with a self-supervised loss. The loss function leverages cross-consistency between the disparities and modalities. In addition, the loss limits the fact that disparities with in the same view should remain zero. The structure of the loss is shown in the below diagram.
Bibtex
@article{gu2022eventintensitystereo,
title={Self-Supervised Intensity-Event Stereo Matching},
author = {Gu, Jinjin and Zhou, Jinan and
Chu, Ringo Sai Wo and Chen, Yan and Zhang, Jiawei
and Cheng, Xuanye and Zhang, Song and Ren, Jimmy S.},
url = {https://arxiv.org/abs/2211.00509},
publisher = {arXiv},
year = {2022}
}
Abstract
Event cameras are novel bio-inspired vision sensors that output pixel-level intensity changes in microsecond accuracy with a high dynamic range and low power consumption. Despite these advantages, event cameras cannot be directly applied to computational imaging tasks due to the inability to obtain high-quality intensity and events simultaneously. This paper aims to connect a standalone event camera and a modern intensity camera so that the applications can take advantage of both two sensors. We establish this connection through a multi-modal stereo matching task. We first convert events to a reconstructed image and extend the existing stereo networks to this multi-modality condition. We propose a self-supervised method to train the multi-modal stereo network without using ground truth disparity data. The structure loss calculated on image gradients is used to enable self-supervised learning on such multi-modal data. Exploiting the internal stereo constraint between views with different modalities, we introduce general stereo loss functions, including disparity cross-consistency loss and internal disparity loss, leading to improved performance and robustness compared to existing approaches. The experiments demonstrate the effectiveness of the proposed method, especially the proposed general stereo loss functions, on both synthetic and real datasets. At last, we shed light on employing the aligned events and intensity images in downstream tasks, e.g., video interpolation application

Result
Results of different methods on synthetic dataset. Monocular model does not work well as it cannot be fine-tuned under this setting. Stereo matching between intensity and reconstruction fails because the color discrepancy prevents the network to relate corresponding pixels. After selfsupervised training, our predictions are on par with the ground truth. The reconstruction network is FireNet and the stereo network is the modified AANet.
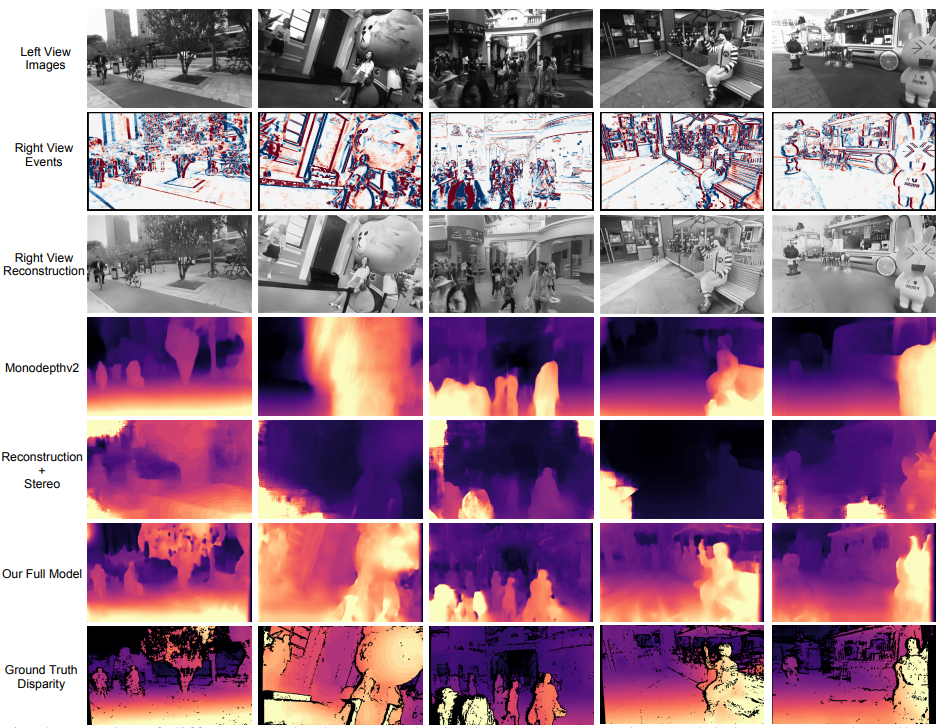
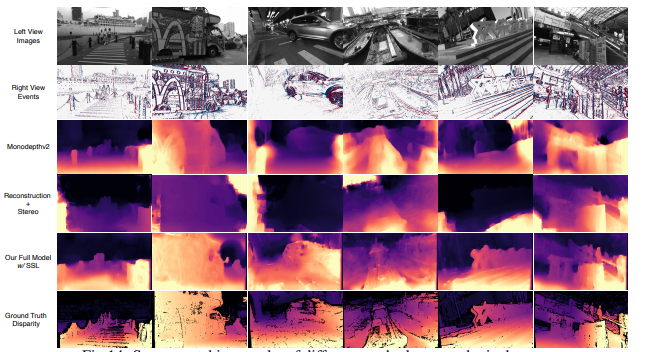
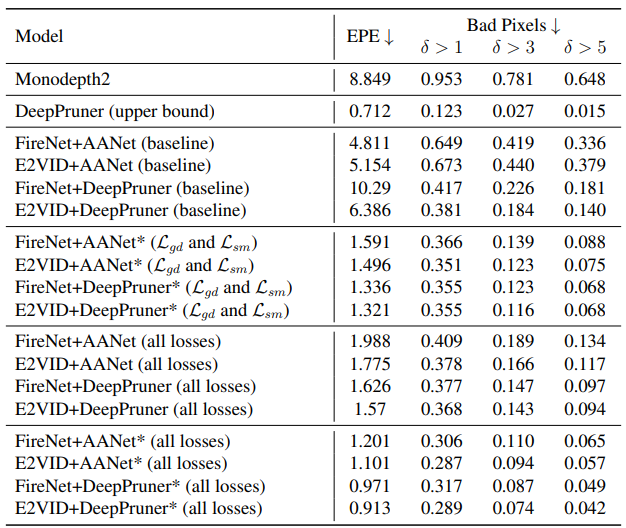
Method
Our self-supervused stereo system follows a few steps: First, we convert event stream to reconstructed images via off-the-shelf models such as FireNet or E2VID, so that we have an image pair, with one intensity image and one reconstructed image. Second, We train the system with a self-supervised loss. The loss function leverages cross-consistency between the disparities and modalities. In addition, the loss limits the fact that disparities with in the same view should remain zero. The structure of the loss is shown in the below diagram.
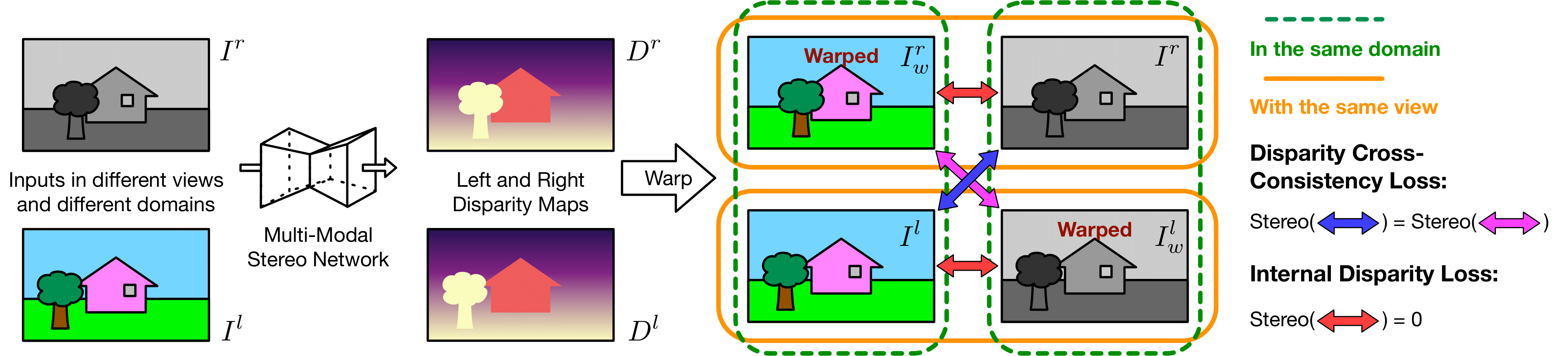
Bibtex
@article{gu2022eventintensitystereo,
title={Self-Supervised Intensity-Event Stereo Matching},
author = {Gu, Jinjin and Zhou, Jinan and
Chu, Ringo Sai Wo and Chen, Yan and Zhang, Jiawei
and Cheng, Xuanye and Zhang, Song and Ren, Jimmy S.},
url = {https://arxiv.org/abs/2211.00509},
publisher = {arXiv},
year = {2022}
}